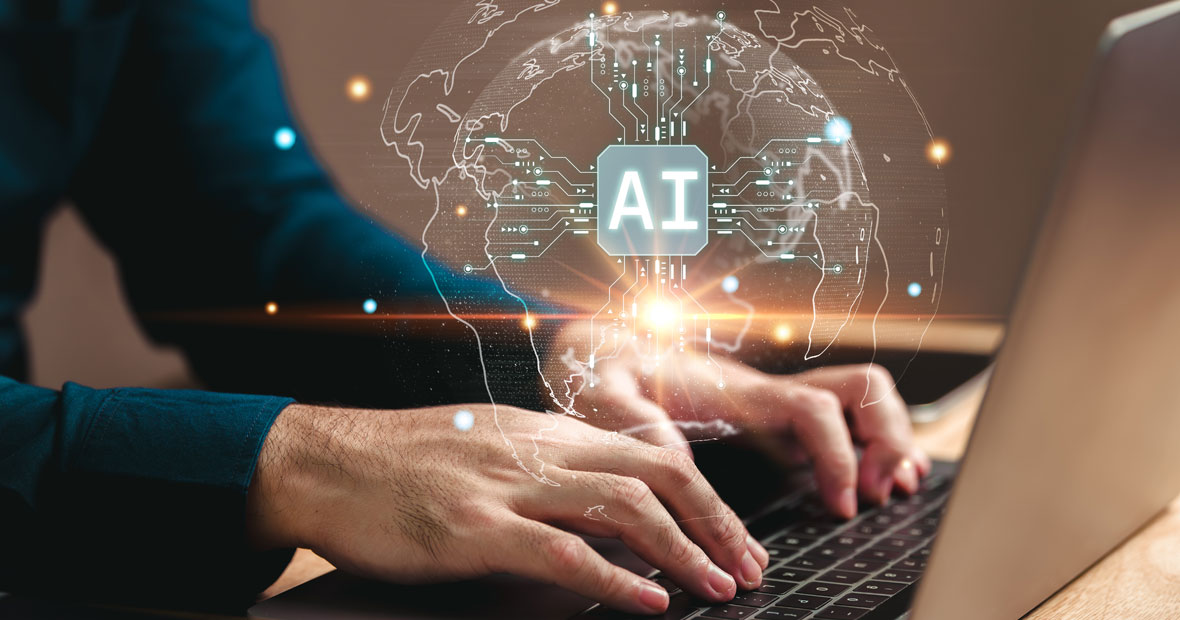
Diving into the realm of artificial intelligence, one quickly realizes the pivotal role played by continuous experimentation and meticulous tracking in crafting the most accurate and efficient AI models. Like seasoned gardeners, AI developers nurture their creations, refining them over time with the invaluable feedback provided by tracking data. This article delves deep into the art of integrating these tracking mechanisms into AI development, shedding light on the transformative power of real-time insights gained from tracking. Further, the narrative unravels how data gathered from tracking can be harnessed to bring about substantial improvements in AI models, propelling them from merely good to the best. Finally, the significance of relentless experimentation in the field of AI is discussed, along with the potential of tracking to turbocharge AI model performance. This journey promises to offer a deeper understanding of the intricate dynamics between tracking and AI model development.
Role of Tracking in Enhancing AI Models
Experimentation mastery introduces tracking as an essential feature for the advancement of AI models. Tracking serves a pivotal role in capturing and analyzing data, which is integral in refining AI models. ML experiment tracking, a specific method, demonstrates a significant impact on AI development.
Integrating Tracking Mechanisms in AI Development
Tracking mechanisms are integrated into AI development processes to monitor and collect data. This data is analyzed and used to fine-tune the AI models, enhancing their performance. The implementation of tracking mechanisms has shown to improve the efficiency of AI models, as evident from several successful case studies.
Real-time Insights from Tracking
The real-time insights obtained from tracking allow for instantaneous adjustments to the AI models. This immediate feedback loop ensures that the models are always optimized, leading to better overall performance.
Improvements in AI Models Using Tracking Data
Tracking data provides valuable insights that can be used to improve AI models. By analyzing this data, developers can identify areas of improvement and make necessary adjustments. This has led to significant advancements in the field of AI, with models becoming more accurate and efficient.
The importance of tracking in AI models is further emphasized by the latest research and technological advancements in the field. While there are challenges and issues faced in tracking, solutions are continually being developed to overcome these hurdles.
Experts in AI have shared their insights on the importance and role of tracking in enhancing AI models. Their experiences are documented in blog articles, providing a wealth of knowledge for others in the field.
Transitioning from Good to the Best AI Model
Transitioning to an advanced AI model is an investment that promises increased accuracy, enhanced efficiency, and swift decision-making. This shift is not without its challenges, yet potential hindrances can be effectively overcome with the right strategies and approaches. By upgrading AI models, numerous companies have successfully enhanced their operations, as evidenced by multiple case studies.
Upgrading to a superior AI model is not a simple task. It requires a series of key actions including identifying potential challenges, testing various methods for effectiveness, and investing in necessary skills and resources. The potential impact on business performance is significant, with the possibility of mitigating risks associated with suboptimal AI models and driving customer satisfaction.
Yet, the transition offers more than just immediate rewards. It allows businesses to stay competitive amidst recent and future AI trends. The cost associated with upgrading may be substantial, however, the potential returns on investment make this a worthwhile endeavor. Continuous learning and training are paramount to staying updated with the latest AI models and technologies. The ethical implications of using more advanced AI models are also worth noting, as they contribute to setting industry standards.
Key points to consider during the transition include:
Effectiveness of different evaluation and testing methods
Required skills and resources
Potential impact on business performance
Best practices for maintaining and optimizing an improved AI model
Costs and potential returns on investment
Necessity of continuous learning and training
Undeniably, the journey from a good to the best AI model is a strategic move that can result in substantial advancements for any organization.
Significance of Continuous Experimentation in AI
Continuous experimentation plays a pivotal role in Artificial Intelligence (AI), paving the way for constant improvement and fostering innovation. It presents an opportunity to promptly identify and rectify mistakes, making AI models more robust and reliable.
Continuous experimentation in AI encompasses a variety of methodologies. It's instrumental in updating AI models and adapting to new data sets. This approach has resulted in noteworthy breakthroughs in the AI field, as evidenced by multiple case studies. However, it's not without its challenges - including potential bias and reliability issues.
Experimentation mastery involves tracking and analyzing the performance of AI models meticulously, a practice that has shown to significantly enhance decision-making and problem-solving abilities. This process requires specific skills and knowledge. Fortunately, numerous educational resources are available for those keen on delving deeper into this aspect of AI.
Businesses and industries worldwide are realizing the implications of continuous experimentation in AI. It's becoming a crucial part of AI development, and its importance can't be overstated. With the help of advanced tools and technologies, organizations are now able to facilitate continuous experimentation in AI more efficiently than ever.
Ethics are a major concern in continuous experimentation in AI. It's paramount to ensure the accuracy and fairness of AI systems, and continuous experimentation provides the means to achieve this. The current and future trends in this field point toward a more ethical and responsible use of AI.
Boosting AI Model Performance through Tracking
Experimentation mastery: How tracking boosts your path to the best AI model
Concreteness to enhancing AI model performance is brought by tracking. Tracking employs various methods, each designed to optimize AI model performance. Real-life instances of improved performance due to tracking are numerous, offering tangible proof of its benefits. Technological tools for performance tracking of AI models abound, aiding in this critical task. Despite potential challenges and hurdles in implementing tracking to improve AI model performance, the benefits often outweigh the difficulties.
Emerging trends and innovations in tracking for AI performance improvement continue to revolutionize the field. A comparison of AI model performance with and without tracking reveals significant disparities, emphasizing the value of tracking. Expert advice and best practices on using tracking for optimizing AI model performance provide valuable insights. Tracking the performance of AI models entails technical aspects, including the use of specific algorithms.
Collecting and analyzing data for tracking AI model performance is essential. This process assists in identifying and rectifying faults within AI models, thereby enhancing overall efficiency. The role of AI in facilitating performance tracking and improving decision-making processes is significant. Implementing tracking to improve AI model performance does come with associated costs, yet these can often be justified by the resultant benefits. Through tracking, transparency and accountability in AI can be enhanced, contributing to a more ethical AI landscape.